Review
The Busara Clinical Decision Making Framework (BCDMF) deliberative phase is designed to be used when decision makers are not ready to make a decision after examining decision-related data and tradeoffs intuitively. Use of the deliberative phase should be considered anytime decisions present difficult tradeoffs and/or when making high stakes decisions, particularly those that cannot be reversed, such as having surgery.
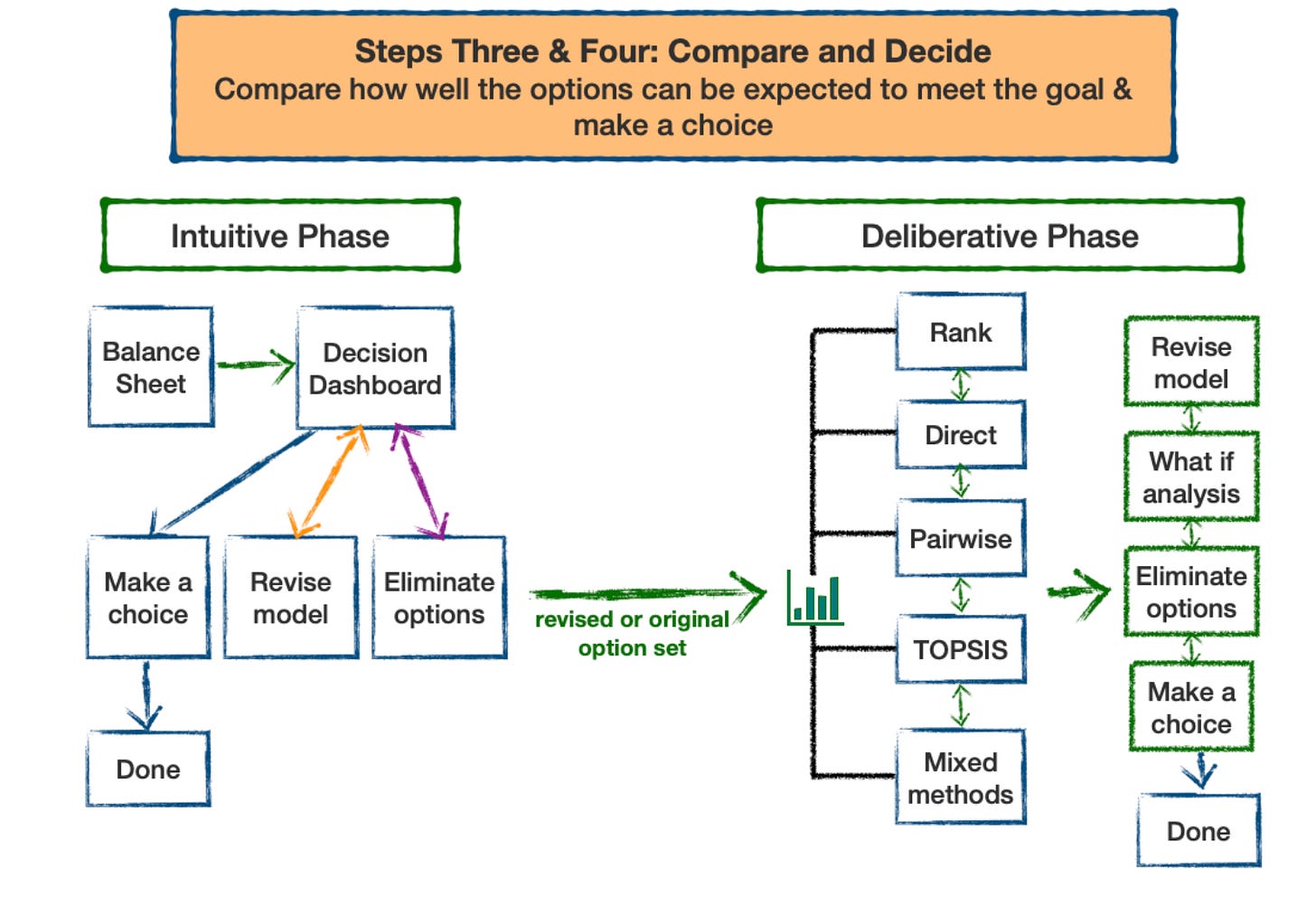
The BCDMF is based on multi-criteria decision analysis (MCDA). MCDA is designed to help people make better choices when decisions involve tradeoffs between competing decision objectives, a characteristic of many medical decisions. There are a number of well developed MCDA methods. They all use the same basic decision model but differ in the method used to identify preferred alternatives. A nice feature is that the methods can progressively build on each other, so it is possible to increase the complexity of an analysis without needing to start over. [1]
The methods included in the Busara Clinical Decision Making Framework are listed in the following table. They can be applied to both assessing the relative priorities of the decision criteria and how well the options meet the criteria.
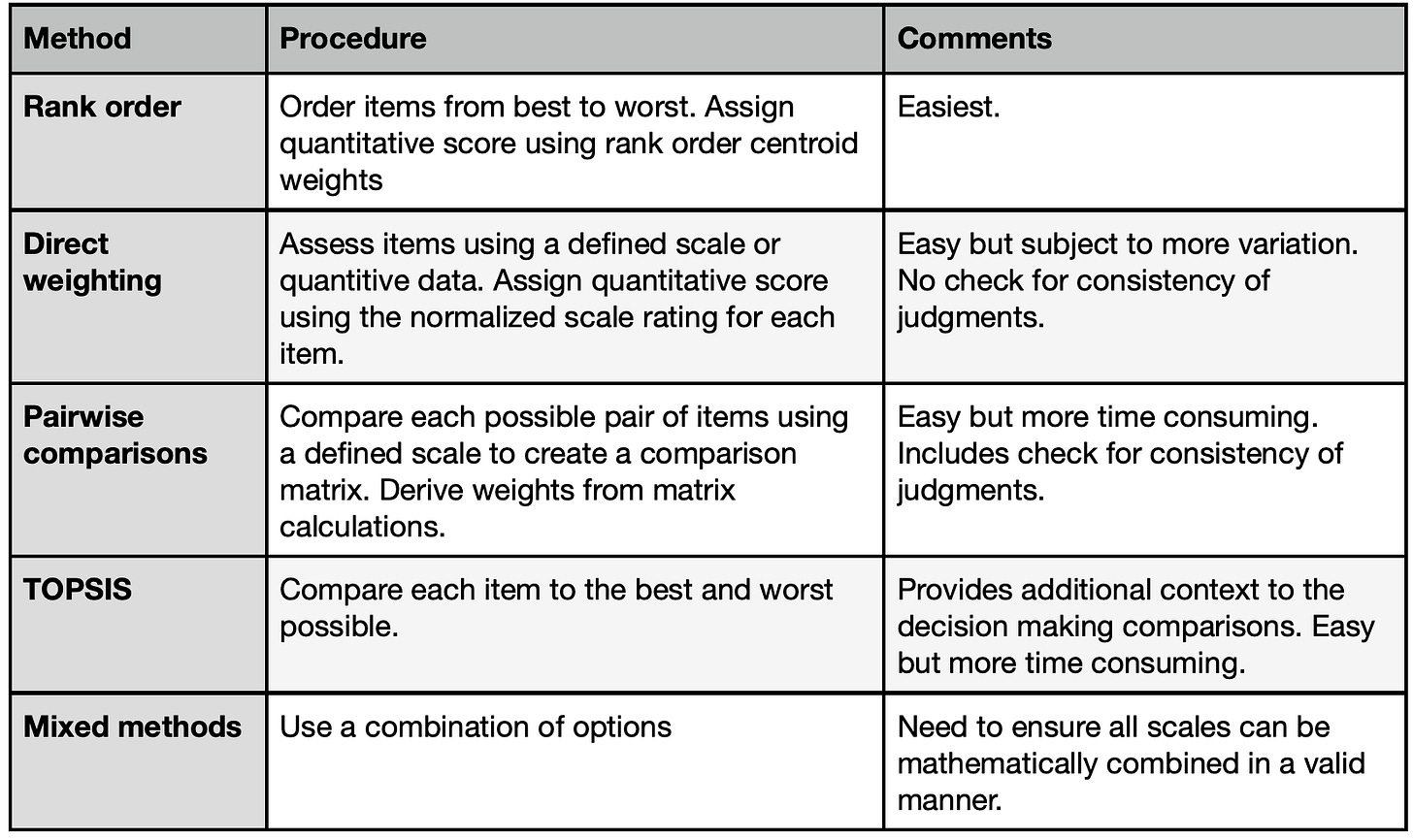
All of these methods work by creating quantitative scales that reflect decision makers judgments about how well the options meet the criteria and the priorities of the criteria relative to the goal. These scales help characterize the judgments being made in the decision making process more exactly than possible using qualitative terms or intuitive feelings. They therefore provide a new and enhanced way for decision makers to communicate with each other about their preferences and priorities. They also enable decision makers to explore how changing their initial preference and priority judgments affects the overall assessments of the options under consideration.
Pairwise Comparisons
The BCDMF pairwise option approach reduces the decision judgments to their simplest form: a single judgment between just two of the decision elements (options or criteria). This approach is used in the Analytic Hierarchy Process (AHP) a well-known decision making method.
The advantage of the pairwise method is that it focuses attention on each individual component of the decision. In doing so, it provides a fundamentally different way of thinking about the tradeoffs and judgments involved in making a decision than the other methods included in the BCDMF (and many other formal decision making techniques). This difference can help decision makers gain additional insight into the decision at hand and help them refine their personal preferences and priorities. The disadvantage of the method is the inevitable increase in the number of discreet judgments that must be made if the decision is broken down so completely.
The benefit of the approach depends on whether the additional insight is worth the additional work involved. Examples include situations where a clear best choice has not emerged after using the other methods provided in the framework and when making a particularly high stakes decision, where the role of a good decision making process is paramount. A good way to use the pairwise comparison format is to minimize the work involved by using the earlier steps in the process to highlight the key features of the decision and identify a short list of options that are worth further in-depth analysis.
To illustrate, let’s continue to use the example scenario where a doctor and a patient named Anna are choosing among 3 possible treatment options using the following decision model.
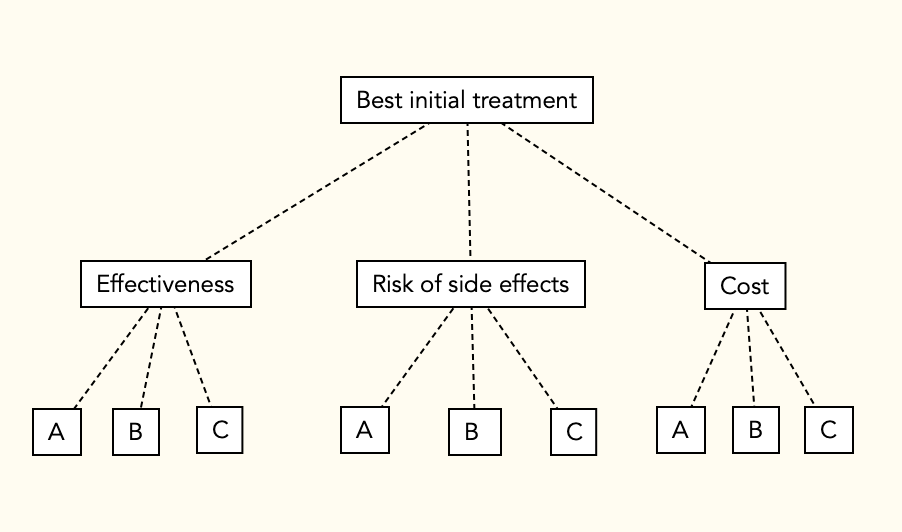
They collect data summarizing how well each option will fulfill each of the three decision criteria:
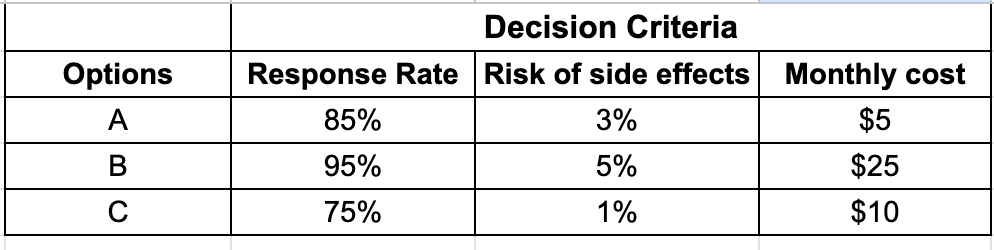
They then evaluate the options and prioritize the criteria using both ordinal rank weights and direct weights, as explained in the last two Musings. The results are summarized below:

Now let’s assume that on the basis of the analysis so far, Anna eliminates Option B from consideration but is still unsure whether she prefers Option A, which is better in terms of effectiveness, or Option C, which is the safer option. She also decides to eliminate the cost criterion, since she can afford both of them equally well. The resulting decision matrix is shown below:
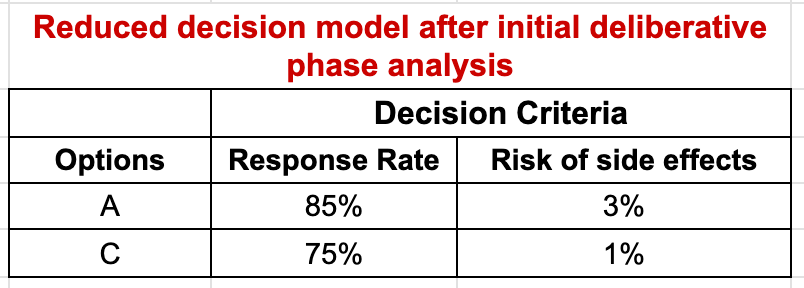
Anna and her physician then decide to use the Pairwise Comparison technique to take a closer look at the differences between these two options. For the judgments regarding how well the options fulfill the criteria, the first step is to decide if the two options are equivalent. If not, the preferred option is identified and the strength of preference judged on a four-point scale: slight (2), moderate (3), strong (4), or very strong (5). These judgments are then entered into a judgment table or matrix. With only two comparisons, this is a 2×2 table. Each row show the relationship between the Row option and the Column option:
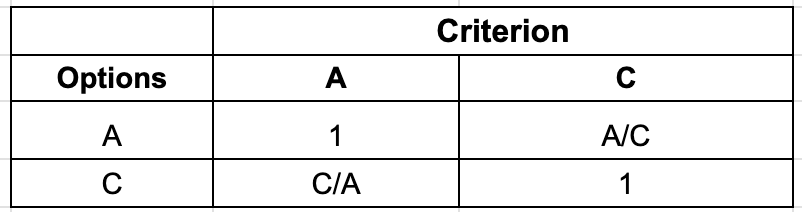
Option scores are calculated by normalizing the geometric means of the row totals. The comparisons between the criteria are made the same way with the judgments made in terms of how important each is relative to the goal of the decision.
The judgments required for Anna’s analysis are summarized below:
- Response Rate: Option A (85%) vs Option C (75%)
- Risk of Side Effects: Option A (3%) vs Option C (1%)
- Response Rate vs Risk of Side Effects relative to the decision goal
Let’s assume that Anna moderately prefers A to C relative to Response Rate, slightly prefers C to A relative to Side Effects, and judges Response Rate moderately more important than risk of Side Effects relative to the decision goal. The resulting comparison tables, geometric means, and scores are shown below:
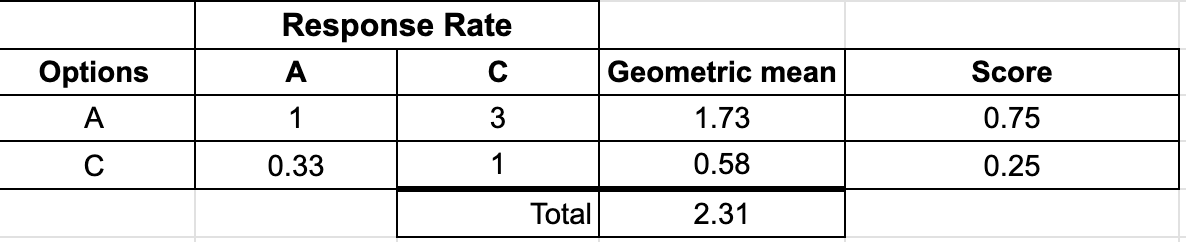
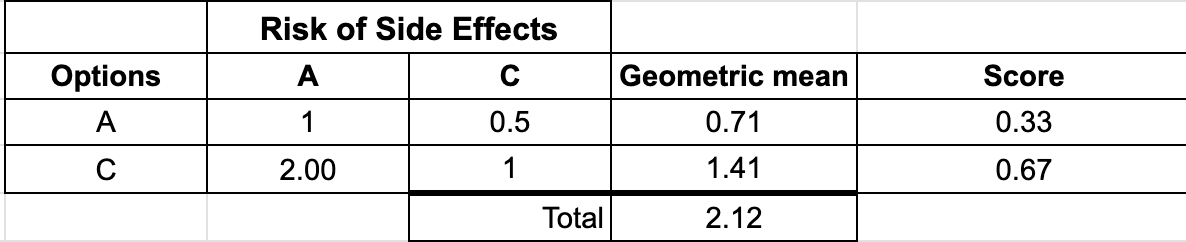
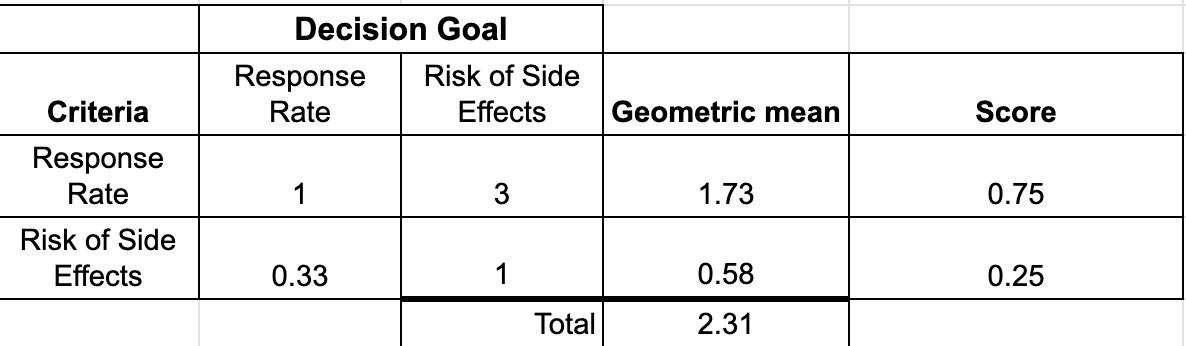
The final results are calculated using the weighed average method, just like the ordinal rank weighting and direct weighting methods: overall scores are calculated for each option by multiplying the option criteria weights times the criteria priorities and summing the results. To make it easier to review and discuss the scores, they are multiplied by 100 to remove the decimal places.
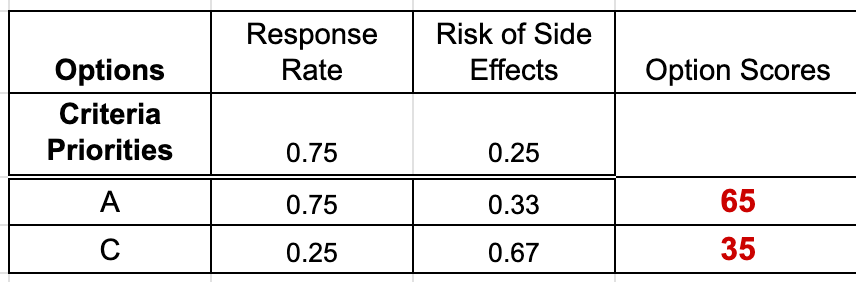
Musings
Although this and the other deliberative methods I have described seem complicated, that is only because I have been explaining how things work “under the hood”. Once the calculations are programmed into a suitable app or spreadsheet, the process only requires attention to the judgments being made.
As I mentioned previously, another advantage of the quantitative deliberative methods is the ability to determine how changes in judgments would affect the final results. The ability to ask “what if” can add a great deal of insight into the key aspects driving a decision.
References
1. Dolan JG. Multi-Criteria Clinical Decision Support: A Primer on the Use of Multiple-Criteria Decision-Making Methods to Promote Evidence-Based, Patient-Centered Healthcare. The Patient: Patient-Centered Outcomes Research. 2010 Dec;3(4):229–48.